Cervical cancer is a significant global health issue, and traditional screening methods like Pap smears are labor intensive and may miss cases. Automation is needed, but it faces challenges in interpretability and data availability. To address this, the paper proposes using Explainable Artificial Intelligence (XAI) techniques like GradCAM, GradCAM++, and LRP to improve the transparency and interpretability of a cervical cell classification model, making it a novel contribution to enhancing the trustworthiness of automated cervical cancer detection. Through VGG16, an accuracy of 91.94% was achieved in classifying cervical cancer cells. The qualitative analysis of XAI methods confirmed that the model relied on nucleus and cytoplasm features, key indicators of malignancy. With the least mean image entropy of 2.4849 and steep prediction confidence drop with perturbations, quantitatively proved Layer-wise Relevance Propagation (LRP) to be the most effective XAI technique for cervical cell classification.
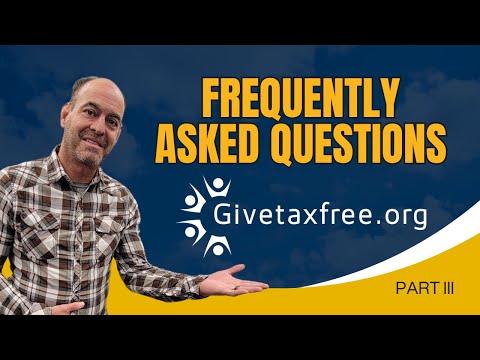
FAQs About GiveTaxFree Answered! PART III
ICARC2024 – Interpretable Cervical Cell Classification A Comparative Analysis [Video]
Categories
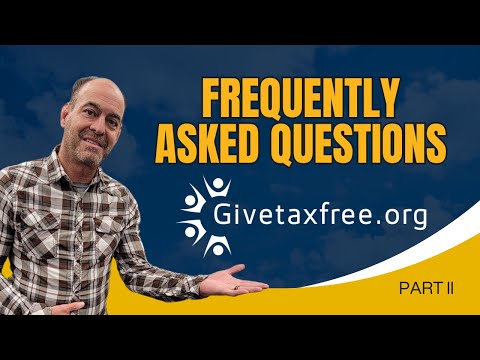
FAQs About GiveTaxFree Answered! PART II